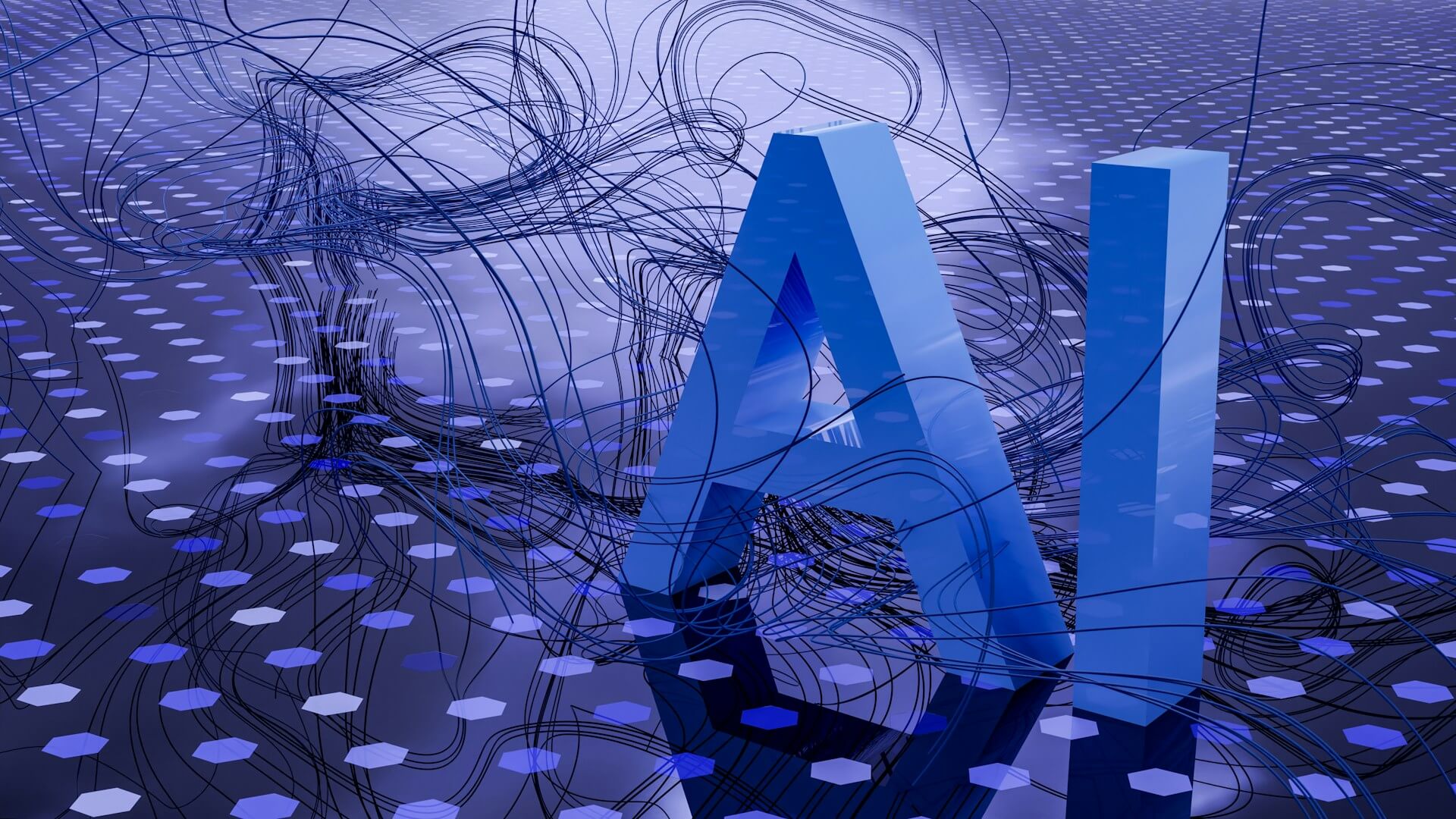
We are in the middle of major discussions about the opportunities and threats of artificial intelligence. Besides economic benefits and prosperity we want good jobs. Research indicates that AI does not automatically lead to good jobs nor to the disappearance of bad jobs: the outcome depends on organisational design and management regimes on the one hand and employee participation in decision-making on the other. This is the struggle for organisational control.
The concept of good jobs means more than wages and permanent contracts; it’s also about work content and labour relations. Theory-based criteria and design approaches for good work are available (Pot, 2023), and policies ought to be following the European Pillar of Social Rights Action Plan, in which the European Commission encourages national authorities and the social partners to foster workplace innovation.
However, the market mechanism does not provide good jobs by itself. Rodrik and Sabel (2019) describe a ‘massive market failure’ to create ‘a good jobs economy’, one example being that the number of workers with monotonous repetitive tasks in Europe did not decrease between 2005 and 2015 (Pot, 2022).
Of course, some routine tasks have been replaced by automation, robots or AI, but German research shows that new repetitive tasks have emerged in their place (Ittermann & Virgillito, 2019; Lager, 2019; Lager et al., 2021). One example is the expansion of the number of warehouses and new technologies such as headphones (audio picking) and Google Glass (vision picking) that lead to higher productivity but also shorter tasks and task intensification; another is Amazon Mechanical Turk that offers workers the freedom to complete very short menial tasks such as recognising and labelling images. Piasna (2024) observed work intensification across all sectors, but most pronounced in construction, manufacturing and healthcare.
To manage the consequences of the digital transition, we need to have a good understanding of the technical and organisational alternatives and the balance of power involved in organisational design.
Organisational control
The employment relationship is not just the legal link between employers and employees regarding work or services carried out in return for remuneration and the presence of reciprocal rights and obligations between the employee and the employer. Marx’s extensive theoretical elaboration explained that technology and organisation play an important role in the struggle over the combination of working time (hours, minutes, breaks) and the intensity of work (effort per hour) in relation to labour productivity and pay (Marx 1887: Chapter 15). Taylor (1911) recognised the struggle for organisational control and tried to solve it by ‘scientific management’ but did not succeed.
Organisational control on the part of management can take different forms: ‘command and control’, or ‘participation and trust’. Informal behaviours can differ as well, for example respect or intimidation. Management, based on algorithms without human intervention, can also include automatic decisions about ratings, rewards and penalties, as we know from the Uber app, and represents a new form of social domination (Nicklich & Pfeiffer, 2023).
Organisational control on the part of workers can also take different forms: task autonomy and skill discretion, autonomous teams and shopfloor consultancy, co-determination and collective bargaining (Lamannis, 2023) or collective action such as strikes. Informal behaviour can either reflect a desire to follow the rules or to try to avoid them, to be proactive or to go slow, and sometimes sabotage. In the context of AI, this boils down to the question of how to fool the algorithm (Cini, 2023). One example is the ‘timed collective logouts by couriers in the twenty-first century that are mirroring the stopping of machines in the twentieth century’ (Vandaele, 2021: 227). Thiel and colleagues (2023) theorise that monitoring paradoxically creates the conditions for more (not less) deviance by diminishing employees’ sense of agency, thereby facilitating moral disengagement via the displacement of responsibility. Zirar (2023) also offers an interesting perspective. In contrast to prior reviews, which generally focus on the apparent transformational strengths of AI in the workplace, his review primarily identifies AI limitations before suggesting that the limitations could also drive innovative work behaviour.
Although the social context has changed considerably, the struggle for organisational control is still ongoing. Perhaps the application of AI will mark the beginning of a new phase of this struggle. The scope for action is vast: Kellogg and colleagues (2020) point out that employers can use algorithms to direct workers by restricting and recommending; evaluate them by recording and rating; and discipline them by rewarding and replacing.
Organisational choice
It is often thought that the appearance of jobs and tasks is determined by technology and by economic factors (efficiency, productivity). However, how work is organised also appears to depend on the chosen management style. This has recently been substantiated with research from the United States. Management practices have at least as much impact on productivity as new technology (Bloom et al., 2019), but they are very different and such differences are difficult to explain. In the organisational sciences, this relativisation of technological and economic determinism has led to the use of the term ‘organisational choice’.
Research on AI: mixed outcomes
Focusing on AI, the same conclusions (about organisational control and organisational choice) can be drawn. Acemoglu and colleagues (2023) point out that artificial intelligence is now mainly used to automate labour, resulting in unemployment and little or no improvement in productivity. An alternative, “human-complementary” path could contribute more to productivity growth and could help reduce economic inequality.
Empirical research confirms that the application of AI can have different effects on job quality. Danish research shows that AI may enhance or augment skills through, for example, the increased use of high-performance work practices, or it may raise the constraints on the pace of work and reduce employee autonomy (Holm & Lorenz, 2021). From 11 case studies across Europe on combined automation and AI systems, Heinold and her team (2023) find, in most cases, work that is less dirty, dull and dangerous in terms of job content while embodying more creative, challenging and cognitive tasks.
According to Wood (2021), the existing evidence suggests that algorithmic management may accelerate and expand precarious fissured employment relations (via outsourcing, franchising, temporary work agencies, labour brokers and digital labour platforms). It may also worsen working conditions by increasing standardisation and by reducing opportunities for discretion and intrinsic skill use. Evidence from platform work and logistics highlights the danger of algorithmic management in intensifying work effort, creating new sources of algorithmic insecurity but also fuelling workplace resistance. Indeed, there may be both positive and negative outcomes for workers, depending on management regime (Kellogg et al., 2020; Poba-Nzaou et al., 2021).
Bérastégui (2021) argues that algorithmic management leads to high job standardisation due to more predictive patterns in the delivery of work and permanent digital surveillance. Platforms are the primary beneficiaries of such practices. This entails, among other things, psychosocial risks. The case of Amazon shows that permanent surveillance not only controls the performance of workers but also their behaviours by countering their attempts at organisational control and curtailing their trade union activities (UNI Global Union, 2021). Furthermore, we know that many recruitment algorithms unintentionally discriminate against particular groups (Burt, 2020).
Another point of contention is the use of AI for increasing occupational safety, some examples of which are known as predictive-based safety, with applications growing in terms of detection and warnings in workplaces and of the use of big data in accidentology and epidemiology. For example, facial recognition may be used to check whether workers are wearing the correct safety equipment. But even then, it has been observed that this can lead to the assessment and disciplining of employees, resulting in workplace stress and mental health problems (Moore & Starren, 2019; Zoomer et al., 2022; INRS, 2023). It turns out to be difficult to experience the advantages of predictive-based safety without the disadvantages of digital control.
Quite a large body of research shows the potential for negative effects in the course of which it could almost be forgotten that AI may also bring about positive innovations in products, services and processes. The benefits for doctors, teachers and judges are also evident where AI supports them to work in a more precise and better-informed way. At the same time, recent research shows that there are significant impacts and risks to the teaching/educating profession such as the solving of tasks by students through various AI-based applications like generative pre-trained transformer (GPT) models (Ghita & Stan, 2022). The influence of these models spans all wage levels, with higher-income jobs potentially facing greater exposure (Eloundou et al., 2023).
Enhancing workplace innovation
Of course, new legislation on AI and labour law reform is necessary and several initiatives at European and national level are underway (Ponce Del Castillo & Naranjo, 2022). However, for organisational control and organisational choice, hard regulation can be supportive, but it is neither sufficient nor particularly effective. In some situations, the joint actions of the social partners and governments provide better opportunities including, for instance, in research and implementation programmes such as Bridges5.0.
Related articles
March 24, 2025
March 23, 2025
March 11, 2025